Bioanalytical labs need to reimagine data to streamline their operations
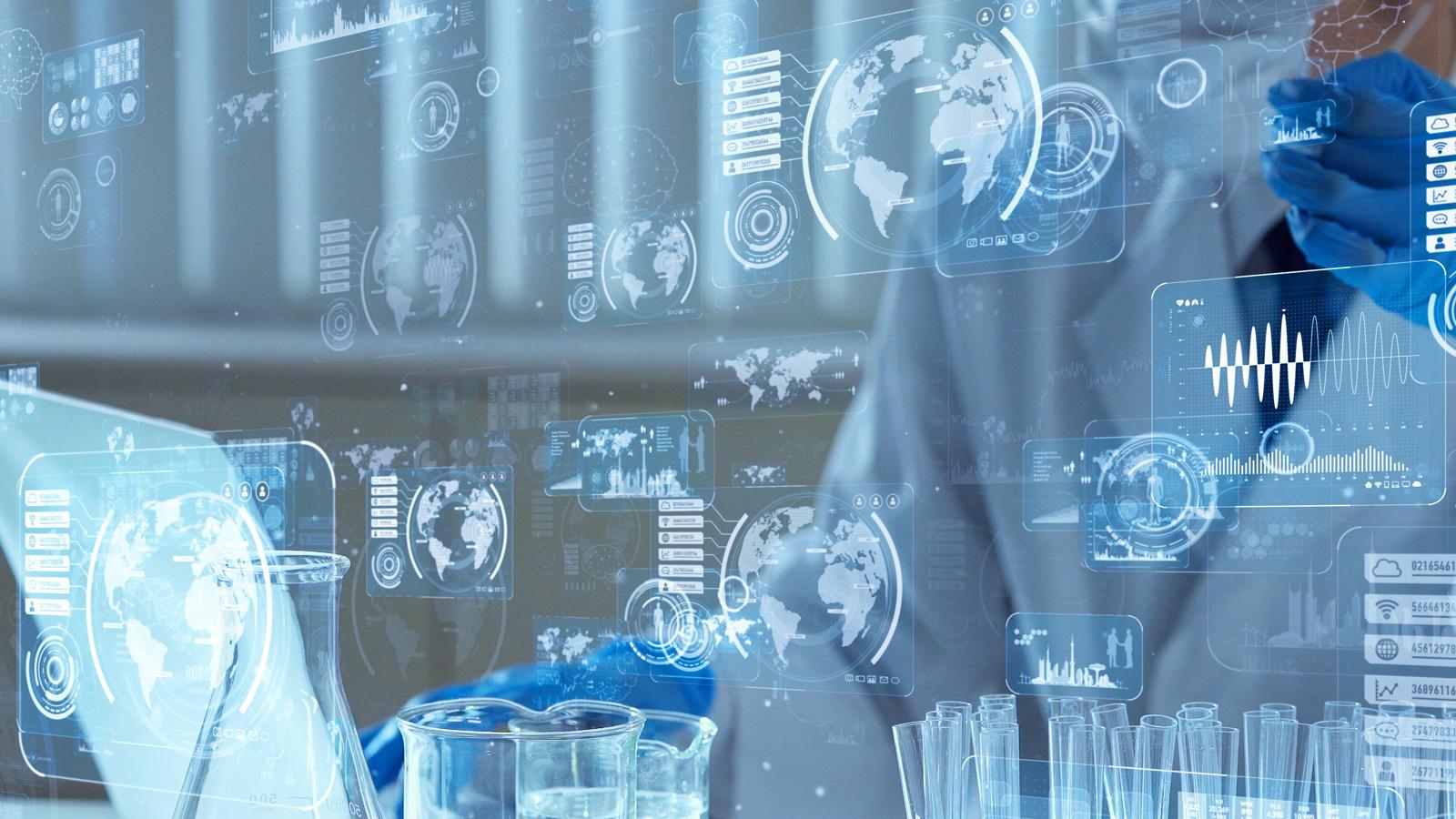
The fundamental questions in the trial phases of drug development are “Does it work?”, “Is it safe?”, and “What else do we need to know?”
These questions can only be answered thanks to bioanalytical labs. The tests that these labs run every day on samples from clinical trials are essential in understanding which drugs and therapeutics will ultimately benefit patients.
The faster scientists are able to make decisions, the faster new drugs can come to market. To facilitate these decisions, scientists need reliable and quality data. Whether labs are housed in sponsor organisations or in contract research organisations (CROs), the underlying challenge is the same: How do we test more samples in less time, without sacrificing accuracy and quality?
Unlocking the data
The answer to that fundamental question lies in unlocking a powerful asset: the data. Smart data management can help labs save time, reduce waste, and provide trustworthy answers more quickly. Better data can not only streamline lab operations and audits; it can also help scientists make faster, better-informed decisions.
In many bioanalytical labs, experimental and operational data lives in too many systems and too many minds. When data is scattered between paper notebooks, spreadsheets, electronic lab notebooks (ELNs), laboratory inventory management systems (LIMSs), analytical instrument software tools, and other siloed systems (not to mention the brains of recently retired colleagues), it cannot be leveraged efficiently. Plenty of actionable, time-saving information slips through the cracks.
For a long time, this status quo was just the way things had to be. But as connected instruments increasingly become the norm, routine laboratory data no longer needs to be manipulated manually – integrated systems can reduce human errors while streamlining operations. And in the era of better cloud storage, more nimble programming approaches, and powerful algorithms, it is also becoming more possible – and necessary – to pull complex experimental data out of historical silos and into a central brain.
Utilising the tools available
This does not have to mean scrapping legacy systems and starting over. Even if data continues to live in an ELN or LIMS, it can now be connected to and made accessible from a centralised data backbone. If data is structured well, with enough meaningful context, the ability to access it from a central location can help to fuel user-friendly reports, workflows, and artificial intelligence (AI) models. With these new tools at their fingertips, scientists can finally unlock the full power of their labs’ data to inform their decision making.
To understand the power of structured data in a bioanalytical lab, consider a scientist developing an assay. This scientist is faced with a new-to-them molecule. But while it is new to them, it may not be new to the lab: perhaps someone else developed an assay for this molecule years ago, then retired. But our scientist does not know that. Instead, they start from scratch. They read about similar molecules, or they stop by a more experienced colleague’s office to chat. They choose assay conditions that they think may work well. They decide how much to dilute their reagents. They try again. Finally, they find something that works. Eureka! They document their process – then save it in a pdf that no one outside their team will ever find or read.
There is, obviously, a better way. Imagine that this scientist’s report – and the reports of their long-retired colleagues – were stored in a central location. This scientist could go back and see which assays were developed, how they were developed, what steps were taken and where those assays were used to support previous studies.
A data management software solution
Imagine, then, if all the experimental data from all the labs’ instruments, samples, and assays over time were also stored and tagged centrally, so that meaningful patterns could appear. Those patterns might not be intelligible to humans – we simply don’t have the time to make sense of them. But generative AI tools can. In the future, it is possible to imagine a system that would be able to provide a useful starting point for an assay, in the same way that ChatGPT can offer a basic outline for an essay or suggest code snippets for programmers.
These approaches require lots of data over time. But labs that build AI-ready systems now, whether to take advantage of generative AI or other machine learning approaches, will soon be able to leapfrog the competition. This new approach to data is a big change, however, and labs that try to do everything at once may get bogged down and disoriented. To be successful, it is better to start small and to build buy-in with visible, incremental wins.
One obvious starting point is to integrate instruments and equipment with a data management software solution. This simple step creates efficiency gains that make everyone’s lives easier, streamlining work for everyone from bench scientists to managers to outside auditors. Imagine the simple but repetitive task of confirming and recording that a balance is calibrated properly before weighing reagents.
Connected and centralised
In many labs, scientists still log this task manually, and whoever is auditing the work must go to the lab to check this step. But with a connected balance, scientists can scan a balance barcode, add the calibration weights and the results will automatically log to the cloud. Reviewers don’t need to check for typos, and regulators can access the information they need with custom digital permissions instead of having to look through messy files or siloed systems. Projects like these can help labs build best practices around structuring data. The ALCOA+ principles can guide labs as they document data: data should be attributable, legible, contemporaneous, original, and accurate; it should also be complete, consistent, enduring, and available. Once data is captured, the FAIR principles can help labs organise it: data should be findable, accessible, interoperable, and reusable.
Next, labs can focus on using connected systems to enforce procedural steps. Common methods can be added to a centralised system and parameters can be set. Anyone repeating the method can do so through a workflow, scanning in the appropriate ingredients as they are used. If someone scans in the incorrect material, the workflow will alert them right away. With fewer micro-decisions to make and fewer repetitive tasks to track, scientists focus their brain power on intellectual challenges and not on busywork.
With the ability to automate exception flagging, organisations can benefit from audit by exception. Instead of taking time to check every detail during analytical run or study review, reviewers and auditors can focus on instances where something out of the ordinary occurred.
Bioanalytical labs that have a strong data management strategy are building strong foundations to streamline their operations. A data strategy should also be defined for experimental data and results. Most of what is reported and published today are results with successful outcomes, but scientists are missing out on a tremendous opportunity to learn from what is unsuccessful in the lab. Soon, AI will help scientists interrogate their data like never before. By working smarter, labs can remain competitive; they can also bring life-saving therapies to market faster.