Researchers use AI to learn 'language' of drug discovery
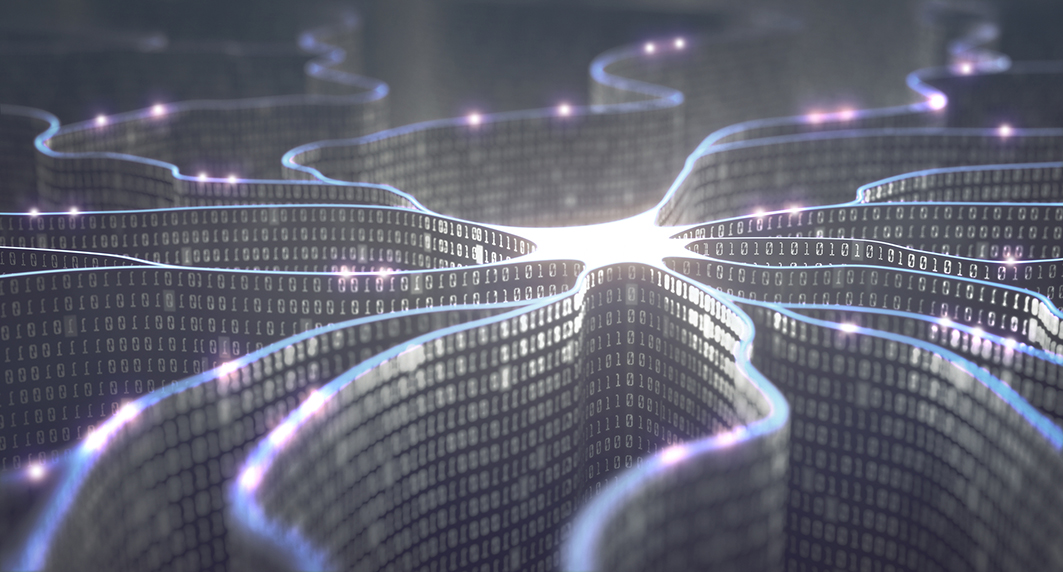
Researchers from Israel are the latest to show that AI and deep learning could make the drug discovery process more efficient, by getting a computer to learn the “vocabulary” of drug discovery.
Increasingly, scientists are turning to computers to remove the element of chance from the drug discovery process.
Whereas in the past new drugs were serendipitously discovered, modern processes begin by screening many molecules and selecting those with the greatest therapeutic potential.
But with more molecules showing potential than there are stars in the galaxy, this is an enormous task.
However in a study presented at the KDD 2018 conference in London this week researchers from Technion - Israel Institute of Technology demonstrated a new approach for generating therapeutic molecule candidates.
The working hypothesis is that drug development has a 'vocabulary' similar to that of a natural language.
The system’s co-developer Shaher Harel said that a system founding on AI and deep learning “acquired” this language based on hundreds of thousands of molecules.
[caption id="attachment_46130" align="alignleft" width="67"] Shaher Harel[/caption]
He added: “We supplied it with the chemical composition of all drugs approved up until 1950, which served as the prototypes upon which it generated new variations – new potential drugs. In order to generate a creative system, we deliberately introduced “noise”, which yields diversity. Namely, the system will generate many variations of existing drugs.”
When they instructed the system to propose 1000 drugs based upon old drugs, the researchers were surprised to discover that 35 of the new drugs generated by the system are existing, FDA-approved drugs developed and approved after 1950.
In other words, the investigators demonstrated the system’s efficiency in developing ‘rational’ or valid drugs.
[caption id="attachment_46131" align="alignleft" width="71"] Kira Radinsky[/caption]
The system’s other co-developer, Dr Kira Radinsky, added: “Instead of seeking out specific correlations based upon hypotheses we formulate, we allow the computer to identify these connections from within a massive sample size, without guidance."
"The computer is not smarter than man, but it can cope with huge amounts of data and find unexpected correlations. This is how we managed to find (in another study) the unknown side effects of various drugs and drug combinations, and now, an innovative drug development mechanism.”
Technion said the breakthrough is particularly important in the light of Eroom’s law, which states that the number of drugs approved by the FDA should decline at a rate of around 50% every nine years.
This is because the ratio between the number of new drugs and the investment in research and development.
The term Eroom was coined in 2012 in an article published in Nature Reviews Drug Discovery, and is a reverse order of Moore, the name of Gordon Moore, one of the founders of Intel. Moore observed that the number of transistors in a dense integrated circuit doubles every two years. In contrast, Eroom’s Law notes that each year, fewer and fewer drugs are marketed.