Machine learning tool aims to cut stress in clinical trial patients
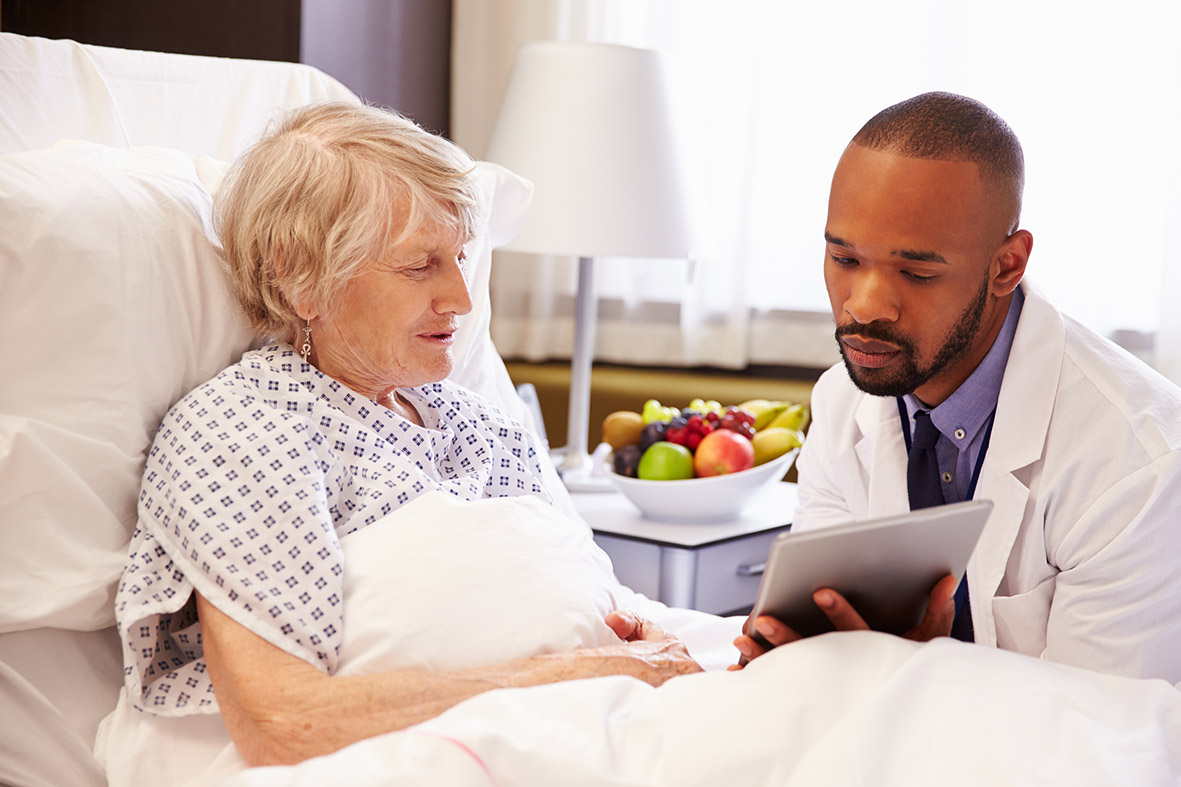
Tech firm Cambridge Consultants has designed a digital health tool that aims to make clinical trials more efficient using machine learning to help prevent patients becoming stressed and dropping out.
The tool known as Verum remotely monitors patients and provides machine learning-driven predictions around conditions or disease states.
Verum’s initial test application focuses on clinical trials, where the company thinks huge efficiencies can be achieved by identifying and mitigating the effect of patient stress on trial outcomes.
Stress is an underlying cause of behavioural and disease states and yet it is poorly understood, leading to badly controlled clinical trials with average drop-out rates at 30%.
For proof of concept, Cambridge Consultants designed a system to measure and monitor a participant’s stress levels during trials.
Developed in consultation with clinical psychiatrists and neuroscientists, Verum uses the power of biometric data, primarily voice and electromyography (EMG), and machine learning to better understand outcomes and increase the likelihood of clinical success.
It said the machine learning-driven system can provide deeper insight on participants during trials through real-time triggers and alerts, enabling clinicians, nurses and trial co-ordinators to mitigate the effect of stress on compliance, investigate stress as a confounding factor and inform better adaptive trial design through continuous data streams.
With the average cost of a phase 2 clinical trial ranging from $7 million to $19.6 million and 80% of all clinical trials failing to reach completion, the company noted that increased efficiency has the potential to deliver very significant savings.
Verum comprises sensors integrated into a wearable, a data collection app and a widget for healthcare dashboards that augments existing data with patient-specific predictions and alerts, for unprecedented clinical insight.
The tool aims to help clinicians, nurses and trial co-ordinators to access biometric and behavioural data from continuously monitored patients, gaining valuable context around a specific condition.
This is achieved by applying a machine learning algorithm to measure patient data, producing a single numerical estimator of a patient’s stress levels at any stage of the trial.
Commenting on Verum, Jaquie Finn, head of digital health at Cambridge Consultants, said: “The rising cost of clinical trials, combined with the commercial risks of failure, mean it’s vital we’re able to harness the power of AI and continuous patient monitoring to mitigate the impact of stress on clinical trial outcomes.”
Potential future applications of Verum include the diagnosis of neurological conditions, the post market surveillance of drugs, the development of closed loop therapeutics, rehabilitation and remote patient monitoring.