The importance of AI literacy in life sciences
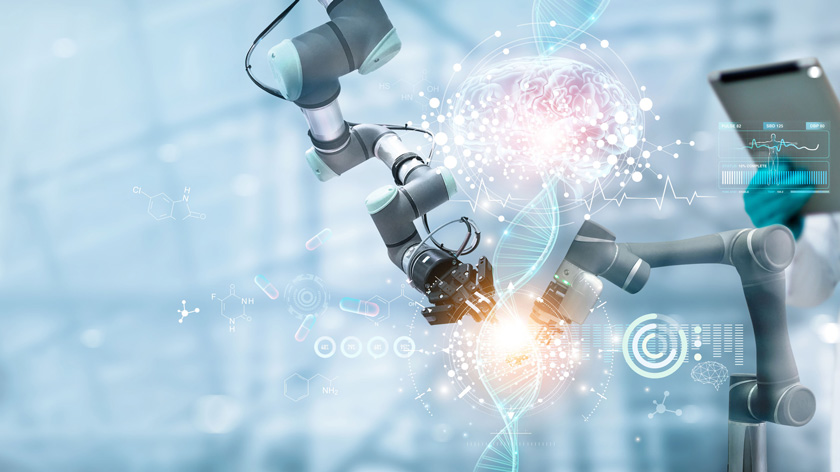
The promise and value of artificial intelligence (AI) in life sciences are somewhat obscured by the over-generalisation of this technology. Indeed, a major misconception surrounding AI is that it’s just tech or some intangible software that we can’t understand, when, in fact, AI itself is a tool for understanding.
For life sciences companies, who must continuously sift through copious amounts of data to decipher actionable insights to inform strategic decisions, AI maximises the time and money invested – driving business efficiencies and positive patient outcomes.
Every company needs to understand what data they are dealing with, what can be done with that data, the models created to understand it, and the real business impact of these combined efforts. To best answer these questions and for business leaders and stakeholders to become more at ease with implementing AI strategies, they need to take a bite-sized approach.
Achieving meaningful adoption rests on shifting public perception from seeing AI as a silver bullet and setting unrealistic expectations for the technology, to looking at it as a strategic tool to deliver incremental, compounding gains.
The understanding gap
Public trust in AI is key to making breakthroughs. Companies delivering therapies rely heavily on patient data, which is pulled through by various means – whether that is direct via their voice delivered through free text, from the data sources they collect, or from anonymised data sets in real-world data analytics.
Given the heavy reliance on data, the life sciences industry must shift the narrative and communicate how this information helps pharmaceutical companies edge closer to creating cures for acute diseases and how exactly that process happens.
The general lack of AI literacy must be addressed by business executives to the users. Further, executives need to understand the technology and its various implications to deliver an achievable strategy. Users need to understand how AI can elevate their ability to deliver patient-centric treatments. Once there is an increased end-to-end understanding, the real business impact will be understood.
This means giving the end consumer a fuller picture of the incremental wins and learnings from failures. As it stands, pharmaceutical companies aren’t celebrating the small wins and doing enough to acknowledge the pressure points, even though this would greatly increase confidence in the technology. Incrementalism is important to this point. Solving massive issues like curing cancer requires small, achievable steps in the right direction. As an industry, we must articulate how companies adopt AI to bring life-saving products to market safer and faster.
An AI developer might, for instance, release a new model once a quarter, which is 3% better than the previous model. At face value, it’s a relatively small improvement, but that 3% could make the difference in discovery because companies will be continually evaluating their data and extracting new insights a year from now. That 3%, compounding with each incremental release, could ultimately unlock a new insight that changes the course of understanding their business.
We should celebrate AI and these wins: that’s how you start convincing people that the technology is worth the investment. As an industry, we need to create a framework for recognising these steps and own the conversation around these incremental wins and how they could ultimately lead to moonshots. Failures, too, must be discussed – regardless of the outcome, there are massive learnings along the way that will improve the next iteration.
Strategising for success
Incrementalism is key for understanding the long-term value of AI, but it is also key for developing longevity in an AI strategy. Where AI adoption struggles is in the understanding of ROI within organisations in which a business strategy positions AI and technology as a blanket solution for every problem or inefficiency. Blanket approaches make it difficult for business executives to measure and understand the return on their investments and, subsequently, they make it difficult to communicate the value to end consumers.
Building for success means taking an incremental approach, celebrating the small wins, and delivering education to all stakeholders at every phase. After all, it’s not about educating just the business executives and product teams. It’s outward-facing – it’s about ensuring that the users leveraging the technology day in and day out can derive value from it.
The companies that have had the most success with AI so far build on a carefully structured and measurable strategy. They’re not looking at AI as a silver bullet, rather they’re considering how they could accelerate portions of their business by applying AI in particular areas. One such example can be seen through Moorfields Eye Hospital’s partnership with DeepMind, in which they trained algorithms, using thousands of anonymised eye scans, to recognise the various signs of eye disease and propose a next step. This endeavour showed that AI made the right referral decision for over 50 diseases with 94% accuracy (equalling the success rate of leading experts). With this technology and an understanding of how to use it, providers can now review scans more quickly and effectively, helping patients to receive the care they need as soon as possible.
The scale, breadth, and volume of the problems companies set out to solve with AI are often too ambiguous and undefined. Businesses struggle when they set out a business strategy and hope to apply AI without a clear understanding of the inherent complexity of the data they are dealing with. The risks further increase when there is a lack of focus on ongoing data collection, cleaning, or consistent access to that data. Healthcare presents a somewhat unique challenge, in that there is so much data from so many different streams that teams need to understand. AI can help, but first, teams need to be able to sift through the noise.
Biotech and pharma companies have good control over their data, working with multiple isolated systems. One of the bigger challenges we’ve worked with at Within3 is getting access to that data in ways that allow us to train effective AI models. That’s done through lots of partnerships with our customers and trying to understand how to bring a data-centric approach to building AI where we’re looking for great data sets that are clean, but not necessarily giant sets of an entire enterprise’s available data.
In reality, so much of the data that pharmaceutical companies are using to train their models is irrelevant. Rather, they need to use the right set of data, which is often a smaller set, if they want the model to understand what specific problem it is trying to solve.
This goes hand in hand with understanding what a company is trying to achieve by applying AI. Artificial intelligence can solve a lot of problems, however, teams must first determine which areas to focus on and the data that will be needed to provide initial alignment between specific business goals and the chosen AI strategy. Without this alignment, investing in data science teams and sophisticated technology can be expensive, and unrewarding.
The law of increasing returns
When used intelligently, AI has incredible potential to accelerate efficiency. Once businesses can apply it to one problem, applying it to the next becomes considerably easier. This bite-sized approach, one where companies set realistic targets, regularly reflect on progress made, and share learnings with stakeholders, not only builds confidence, but also prevents mistakes.
Being realistic about AI applications, which comes from understanding what AI is here and what it can do, will elevate the ability of life sciences companies to make the most of the data at their disposal and get products onto the market faster and safer.
About the author
Jason Smith, chief technology officer, AI & Analytics, joined Within3 in April 2021 through the acquisition of rMark Bio, where he was the co-founder and CEO. Jason began his career at IBM and ATI Research, while studying computer science at Harvard University. He was recruited out of school to the west coast, where he became a serial entrepreneur in the fields of video encryption, high-performance computing, and bioinformatics. Jason served as the VP of Corporate Development at Seattle-based Venture Studio, BE Labs. While at BE Labs, he led investment and development of multiple startups in areas of social networking, data analytics, distributed systems, and consumer experiences. After BE Labs, Jason moved to Chicago to launch rMark Bio – an AI platform for the life science industry.