AI in Drug Discovery: Day Two
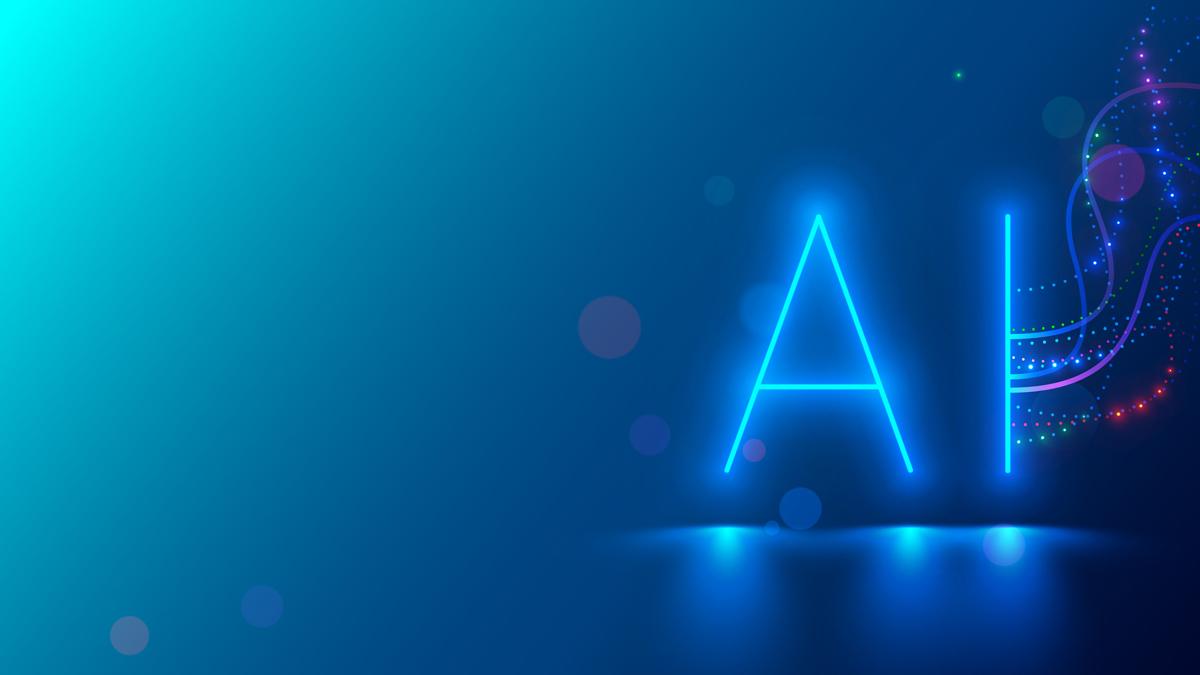
Back in the picturesque West End of London, even a sudden, torrential downpour could not dampen the spirits on 12th March as speakers and attendees returned once more to the Hilton Hotel in Hammersmith for Day Two of AI in Drug Discovery.
Building on the foundation of computational chemistry, machine learning (ML), and large language models (LLMs) discussed on Day One, the agenda featured an array of esteemed speakers from across the field. Here, we break down the key highlights from the event, from a collaborative “Facebook for drug discovery” to the promise of using automated robots to deliver consistent and replicable research outcomes.
Quantum chemistry, deep learning, and AI-physics hybrid approaches
Unfortunately, weather-related transport issues meant that the first two presentations - the first delivered by Dr Christina Schindler, associate director and head of computational drug design at Merck (MSD in Europe), on her team’s efforts to build a sustainable AI and 3D modelling platform to aid hit identification and lead optimisation for small molecule therapeutics - had already passed by the time pharmaphorum made it to the conference room. The microphone had instead been passed to Professor Dr Michael Edmund Beck, a distinguished science fellow at Bayer.
He began by providing an overview of quantum chemistry, encompassing the modelling of molecular properties and reactions, before delving into the synergistic potential of combining first-principles quantum mechanical simulations with data-driven ML approaches.
Next joining the room, via a somewhat tenuous Zoom link, was Dr Haruna Iwaoka, senior director of advanced modelling and display at Discovery Intelligence, Astellas Pharma. But, though the connection may have been shaky, her presentation showcased a solid foundation of success in leveraging the power of deep learning analysis combined with advanced software and automation technologies.
Iwaoka began with a brief background of the Mahol-A-Ba platform, which, she explained, established a “Human-in-the-Loop” drug discovery process that integrates humans, AI, and robots. Consequently, Astellas has significantly reduced the time it takes from hit compound to acquisition of a drug candidate compound. Having set the scene, she highlighted a collaboration between Astellas and Yokogawa Electric Corporation, through which researchers have leveraged the power of deep learning analysis combined with advanced software and automation technologies to study induced pluripotent stem cell (iPSC) differentiation using live-cell imaging data. Using the collaboration as just one key example, Dr Iwaoka underscored the versatility of this technology in facilitating atypical experiments, including assay development, thereby broadening the horizon for scientific discovery.
To follow was the only dual speaker presentation of the event, with BIOVIA’s Dr Ceren Tuzmen Walker and Dr Anne Goupil-Lamy taking to the stage to discuss the company’s efforts in developing tools that blend deep learning with physics-based approaches.
Costly and time-consuming bottlenecks in the drug discovery process are an ongoing challenge for researchers, explained Dr Walker, but with AI tools, we can identify the best candidates, which ultimately improves efficiency. Focusing on BIOVIA’s collaborative SaaS environment, which she described as a “Facebook for drug discovery”, Dr Walker highlighted the platform’s scalability and ease of use, illustrated through a use case involving a partnership with the Ontario Institute for Cancer Research.
During the partnership, she explained, extensive in-house data was used to train models and identify kinase targets promoting YAP/TAZ-driven tumour growth. After several iterations, hundreds of compounds were reviewed, with ten options synthesised and seven validated in vitro.
Taking over from Dr Walker, Dr Anne Goupil-Lamy turned the discussion to the importance of target characterisation in both small molecule and biologics development. Traditionally, she explained, a multi-model approach has been used, but there are cases where homology modelling doesn’t work. To address this, the team decided to join the OpenFold consortium and utilise AI models like AlphaFold2 for target characterisation.
In the context of understanding long COVID, the team modelled nucleoprotein N as a potential target. While initial models showed no binding site, OpenFold revealed the protein’s flexibility and enabled the identification of a large binding site and two nucleopeptides that could bind. Subsequently, the team focused on finding small-molecule options.
Creating consistency with automation
After a brief coffee and catch-up break, it was time to head back into the main presentation room to begin the next round of discussions. The first to bat was Kinga Bercsenyi, vice president of business development at Arctoris.
Bercsenyi began by painting a vivid image of the current state of drug discovery: a cycle often spanning five years to transition from a target to a clinical candidate. Traditionally, she explained, researchers have primarily associated lab automation with high-throughput screening applications for hit identification. However, the rise of AI/ ML for drug discovery has put a clear and increasing focus on the need for improved data depth and data quality, a trend that Bercsenyi noted could open doors for a model where robotic automation and AI not only coexist, but thrive together, enhancing the drug discovery process.
Arctoris is not in the business of replacing scientists with machines; instead, the company leverages robotic arms and automation systems to generate robust clinical data, thereby minimising the cycles required for discovery and development. This approach addresses the “huge data challenge”, focusing on reducing heterogeneous datasets riddled with errors and improving metadata capture.
A critical takeaway from Bercsenyi’s presentation was the importance of assay robustness and reproducibility. She emphasised the point: “If you put chemistry into an assay that is not robust, it doesn’t matter how good the chemistry is; it will fail.”
One of the more light-hearted, yet illustrative, moments came when Bercsenyi discussed the seemingly mundane task of shaking a plate in the lab, pointing out how robotics and AI ensure consistency in such tasks, leading to reliable outcomes. This “ecosystem of excellence”, as she termed it, hinges on precision in both action and data collection, transforming scientists from technicians into innovators who can focus on the essence of their research.
Arctoris’s vision transcends the role of a traditional Contract Research Organisation (CRO). By shifting from “big data” to “good data”, the company aims to optimise the drug discovery process. Bercsenyi concluded with a poignantly humorous note on the humane aspects of automation: “No humans were harmed in the making of this data, and no one had to work at 2 AM on a Sunday.”
Echoing Bercsenyi’s opening remarks, the next speaker, Dr Rabia Khan, founder and CEO of Serna.bio, emphasised the importance of data during her presentation, which focused on leveraging the untapped potential of the human transcriptome to revolutionise drug discovery.
Her journey into this new frontier began with the critical task of constructing an accurate and comprehensive dataset - an essential foundation for any valid AI-driven analysis, she explained. Employing ML methodologies, Serna.bio has developed a platform capable of navigating the complex landscape of the human transcriptome, setting the stage for significant advancements in drug discovery.
Drawing from ML, Dr Khan shared the development of the StaR rules – principles derived from extensive analysis, which guide identifying and evaluating potential drug targets within the RNA universe. These rules, she said, underscore the shift towards targeting RNA, a realm rich with classically undruggable proteins and previously unexplored therapeutic opportunities.
Following this, Dr Khan highlighted the immense financial investment in drug discovery, which surpasses the entire GDP of nations like Qatar, underscoring the industry’s urgent need for more efficient and innovative approaches. Reflecting on an AstraZeneca review, she pointed out that a significant portion of pipeline failures between 2005 and 2010 were due to safety concerns, indicating the industry's fundamental misunderstanding of biology.
“We are not looking in the right place, and we don’t have the tools to even begin to look for the right place,” Dr Khan stated, emphasising the importance of understanding how DNA changes translate into RNA variations and, ultimately, affect protein function.
One of her most critical insights is the need for a comprehensive ontological framework for RNA, akin to what exists for proteins. This involves mapping RNA structure, function, and relationships at scale – a daunting task, given the traditionally small datasets available for RNA. However, Dr Khan’s team has made significant strides, spending three years creating large datasets that reveal RNA’s potential for drug discovery.
“It’s easy to find a small molecule that binds to RNA; it’s hard to find one that binds to RNA and elicits a lasting outcome,” Dr Khan explained, highlighting the complexity of achieving functional outcomes through RNA targeting.
With the presentation concluded, Bercsenyi and Dr Darren Green rejoined the stage to begin a dedicated Q&A session on the nuances of utilising data generation and machine learning in drug discovery.
The first question went to Dr Khan, as an audience member enquired about the paradox of needing more data for better results. Putting her scientific hat on, as she put it, Dr Khan highlighted Serna.bio’s strategy of partnering with pharmaceutical companies, acknowledging the challenge in attracting investors to platform technologies. This pragmatic approach underscores the essential role of comprehensive data in advancing drug discovery, despite the apparent counter-intuitiveness.
The conversation shifted to the differences between RNA and proteins, with Dr Khan cautioning the audience to take her computing perspective with a grain of salt, joking, “I’m a biologist.” She acknowledged an overlap between protein and RNA binders, suggesting that the scientific community might not have explored these interactions broadly or deeply enough. This perspective was reinforced by Dr Green’s comment on the serendipity often experienced in research, where shifting focus can unexpectedly illuminate new findings.
When asked how to avoid producing highly reproducible but irrelevant data, Bercsenyi addressed the challenge with an anecdote about an organoid that failed to grow due to inconsistent experimental conditions, illustrating the importance of standardisation in laboratory processes.
Finally, both speakers offered their advice for engaging pharmaceutical companies in new drug discovery approaches. Dr Khan revealed that starting small and demonstrating value with challenging targets can be an effective strategy. This approach, she said, led to a successful partnership for Serna.bio, showcasing their capability to drug the “undruggable”.
Bercsenyi echoed the sentiment on the limitations of human precision in lab settings, noting the impracticality of manual pipetting at nanolitre scales every few seconds, thereby highlighting the indispensable role of automation and AI in modern drug discovery.
In the final pre-networking lunch presentation, Dr Quentin Perron, co-founder and CSO of Iktos, discussed the integration of generative AI (GenAI) and structural information to accelerate the drug discovery process, from hit discovery to lead optimisation.
He opened by explaining the difference between automated and autonomous systems. Automated systems, he explains, operate within a well-defined set of parameters, which means that they are very restricted in what tasks they can perform. In contrast, autonomous systems leverage AI to learn and adapt to a dynamic environment, allowing it to act with limited human oversight and intervention.
The talk highlighted the use of AI-driven retrosynthesis with robotic constraints, enabling the seamless transition from virtual molecules to robotic systems for streamlining design-make-test-analyse (DMTA) cycles. Iktos’ platform, Makya, leverages GenAI to design new and innovative molecules by integrating numerous constraints to automatically generate optimal candidates.
Dr Perron discussed the advantages of template based GenAI, which offers a fast and efficient approach to molecular design. However, translating plain text into instructions for robotic systems requires careful consideration, as blindly trusting patent protocols may not be advisable. To address this challenge, he explained that Iktos has developed its own chemistry that is compatible with robotic systems.
For each reaction in their workflow, the company has generated reaction templates. When designing a new molecule, the planning algorithm matches the target structure to the most suitable synthetic route. After human review, the ordered synthesis instructions are executed by the platform.
Relationships: Who you gonna call?
Kicking off the afternoon’s sessions, Dr Thierry Dorval, head of data sciences and data management at Servier Pharmaceuticals, focused his presentation on using knowledge graphs to address the current weaknesses in approaches to small molecule screening.
Although not a chemist or biologist by training, Dr Dorval explained that he collaborates closely with experts in these fields. Spotlighting these collaborations, his discussion was underpinned by the importance of “relationships” in understanding and leveraging the available data. One use case explored was the dynamic design of focused screening libraries, leveraging knowledge graphs to curate and refine the compound selection process.
Building upon this idea, Dr Dorval emphasised the goal of creating knowledge from the screening process itself, rather than treating it as a simple data generation exercise. The automated design of focused libraries was presented as a means to leverage the power of AI to derive insights and drive more efficient screening campaigns. “I want to benefit from what the AI can provide,” he concluded.
The second of Servier Pharmaceuticals’ double bill of presentations starred one Dr François-Xavier Blaudin de Thé, who was tasked with discussing the company’s AI-powered platform, Patrimony.
He explained that the platform serves three main purposes: target prioritisation, drug repurposing, and drug combinations. To illustrate its capability, Dr Blaudin de Thé detailed Servier’s target prioritisation approach for Amyotrophic Lateral Sclerosis (ALS), which combines two models: Patrimony, an internal platform that builds knowledge graphs from disease-specific data, and Drug Target ID, a collaboration that identifies key biological processes and scores genes for relevance and drugability.
Regarding target scoring, the platform considers two factors: the target itself and its link with ALS. Dr Blaudin de Thé explained that genes important for a disease tend to cluster on a protein-protein network, forming a pathway relevant to the disease of interest.
Once a list of potential targets is generated, the team delves deeper into the biology by modelling the disease of interest with transcriptomic data. Even after identifying relevant targets for ALS and understanding their significance, the team must assess their drugability, leading to the development of an early target assessment tool.
Martin Buttenchoen, a research student with Oxford Protein Informatics Group at the University of Oxford, kicked off his presentation with a light-hearted reference to a 1990s TV show, coining the term “Pose Busters” to set the stage for an in-depth exploration of the intricacies of docking models in small molecule docking. He structured his talk into two main parts: mutations of docking models (the “Pose Busters” talk) and biases in binding affinity work done by his colleague, Guy Durant.
In the first part of his talk, Buttenchoen clarified the use of root mean square deviation (RMSD) as a standard metric for evaluating docking models, mentioning a threshold of two angstroms RMSD considered valid for a pose’s accuracy. However, he presented visual examples to demonstrate the failure modes of docking predictions, highlighting instances where chemically implausible structures, like pretzels, were predicted. This showcased the need for better validation metrics beyond RMSD alone. To address this issue, Pose Busters was introduced as a toolkit designed to identify and correct these failure modes, ensuring chemical validity, geometrical consistency, and intermolecular validity in the predictions.
The second part of the presentation discussed the biases present in binding affinity predictions, emphasising the importance of recognising and correcting these biases to enhance the reliability of docking models.
Buttenchoen then detailed a case study evaluating various docking tools, including classical methods like AutoDock Vina and GOLD, as well as machine learning-based methods, such as DeepDock and Unimog. The study aimed to compare these tools on the ASEdb test set, assessing their accuracy based on RMSD and the physical plausibility of the poses using Pose Busters.
Following Buttenchoen was VP of AI for BenevolentAI Dr Nicola Richmond, who began by giving an overview of BenevolentAI, a clinical-stage biotech company leveraging AI to understand complex diseases and discover novel treatments. The company emphasises patient-first approaches, scientific rigor, safety, privacy, minimising algorithmic bias, and prioritising explainability in AI models, she explained.
Moving onto what she called the “prophet of doom”, Dr Richmond highlighted the challenges in drug discovery, stating, “discovering and developing medicines is really hard. It costs a lot of money, it takes forever, and we fail most of the time.” She argued that a key reason for the high failure rate is often a poor understanding of disease biology, particularly in the selection of drug targets.
To address this, she explained that BenevolentAI adopts a systematic approach to target identification, combining multimodal biomedical data with AI tools. The company has seen successes in collaborations with AstraZeneca, repurposing opportunities for Eli Lilly drugs during COVID-19, and developing an internal pipeline of novel treatments.
She introduced a new modelling framework focused on explainability, aiming to augment rather than replace human scientists. The framework facilitates a systematic process for discovering targets, starting with a biologically driven question, using AI models to rank the protein-coding genome, and then assessing targets for biological plausibility and other criteria.
Also spotlighted during her talk was the enriched sequential learning (ESL) framework, consisting of two transformer-based models: the “Retriever” and the “Reasoner”. This framework allows for evidence-based reasoning over the biomedical literature and structured data, providing a dynamic and explainable approach to target identification.
She concluded by showcasing the performance of the ESL framework against internal benchmarks and its ability to predict clinical trial successes. The framework outperformed genetic evidence in ranking clinical trial outcomes, highlighting its potential to significantly impact the drug discovery process.
Before the final comfort break of the day, it was time for Dr Guglielmo Iozzia, associate director of Data Science, ML/AI, and Computer Vision at MSD Ireland to discuss the limits of ChatGPT and generic large language models (LLMs) for drug discovery applications, as well as strategies for transforming LLM technology to create more effective solutions in this domain.
“The moment you move to production, the first question is: How much are you spending for this?” he began, highlighting the financial constraints that often hinder the adoption of new data-driven technologies. “You think it’s hard to talk to scientists,” he joked. “Try budgets!”
Turning his attention to LLMs, he delved into the specific limitations and challenges of generic models like ChatGPT in the context of drug discovery, citing a need for: generating highly specialised and domain-specific outputs; the tendency of LLMs to generate plausible-sounding, but factually incorrect, responses – also known as hallucinations – which can be problematic in scientific domains; limited context compression; and the requirement for specialised training data from the drug discovery domain to fine-tune and adapt LLMs effectively.
Dr Iozzia emphasised the potential benefits of building, training, and scaling bespoke models tailored for drug discovery, despite the associated investment. He suggested that, while the initial training costs may be significant, the costliest aspect is often the development phase, which includes data curation, model architecture selection, and fine-tuning.
Furthermore, he discussed the challenges of deploying and inferencing successful models, as the computational requirements and associated costs can escalate rapidly with the model’s popularity and usage.
To address these challenges, Dr Iozzia proposed exploring alternative approaches to fine-tuning and deploying LLMs, such as modifying the behaviour of open-source LLMs using domain-specific retraining, supervised fine-tuning, leveraging open-source frameworks like OpenIntel, ONNX, and FFML for efficient deployment and inference, analysing the trade-offs between model size, computational requirements, and performance for in-house models, and investigating cost-effective alternatives to GPU-based inference, such as leveraging RAM for cheaper inference or utilising frameworks like Microsoft DeepSpeed and Hugging Face’s Accelerate for more efficient deployment.
Domain-specific models vs LLMs
With the final break of the event now complete, it was time for the last two talks of the day.
First up to the helm was Dr Iain Moal, scientific leader and GSK fellow in computational antibody engineering at GSK Research & Development, who began by highlighting the advantages of domain-specific models over generic LLMs for tasks such as antibody engineering. He emphasised the need for tailored utilities and accurate output in this specialised field.
Finally, it was time for Marwin Segler, principal researcher at Microsoft, to close out the day’s presentations. Despite being called up to replace a colleague who was unable to make the event, Segler made the stage his own as he discussed the application of generative and predictive models to efficiently explore chemical space, addressing the challenges of data sparsity and the combination of diverse machine learning models in the context of drug discovery projects.
Segler began by acknowledging the increased understanding and awareness of generative AI among the broader audience, thanks to the popularity of systems like ChatGPT. He highlighted that the field of chemistry has been leveraging generative approaches for a long time, citing examples such as generative chemistry and AI-driven de novo design.
Moving on to the present landscape, Segler discussed specialised generative models for molecules, emphasising the need to navigate the vast chemical spaces efficiently without missing potential “hotspots”.
“The question is how can we fit this sweet spot with our algorithms and give us molecules that we can access in a reasonable amount of time and reasonable synthetic effort, while exploring the largest possible chemical space for a problem at hand, which can also be very different from project to project?” he explained.
One of the projects Segler discussed involved a collaboration between Novartis and Microsoft, focusing on generative chemistry. The project followed a cycle of generation, post-processing, and selection for synthesis. While unable to share specific details, Segler mentioned that some of the molecules generated by the models were initially met with scepticism from chemists, prompting the team to seek chemists’ input in ranking the generated molecules.
Looking towards the future, Segler highlighted the potential of LLMs in generative chemistry. He jokingly acknowledged the positive impact of discussing LLM for the company – “Use more [LLMs], it drives up the Microsoft stock price!” – underscoring the widespread interest and excitement surrounding this technology.