The third wave of AI in pharma R&D
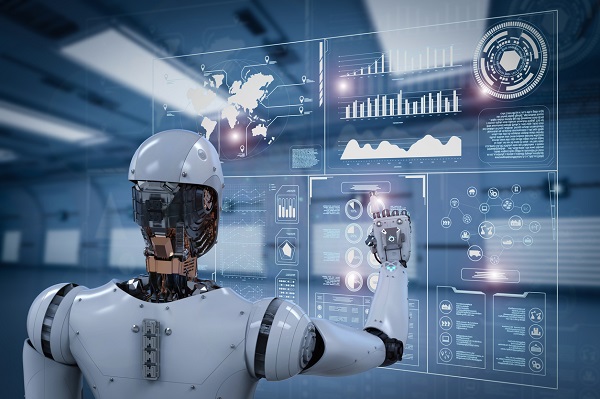
The capabilities of artificial intelligence are advancing and its 'third wave' offers the ability to analyse enormous sets of data, identify patterns, and generate algorithms to explain them, to the benefit of researchers.
The digital revolution vastly accelerated the research, development, and production of new drugs. Digital technology has augmented the natural capabilities of researchers and scientists in a variety of ways. Now, artificial intelligence (AI) is poised to take this augmentation to the next level.
One of the most significant ways in which AI technology augments human capacity – particularly in an R&D context – is by automating repetitive, lower-level cognitive functions that once had to be carried out manually. This liberates drug researchers to focus on higher-level thinking. This advantage was identified early in the development of AI technology by J C R Licklider, who wrote back in 1960 in Man-Computer Symbiosis:
“About 85% of my ‘thinking’ time was spent getting into a position to think, to make a decision, to learn something I needed to know. Much more time went into finding or obtaining information than into digesting it.”… “Several hours of calculating were required to get the data into comparable form. When they were in comparable form, it took only a few seconds to determine what I needed to know.”
A related idea was expressed by Herbert A Simon with the concept of ‘bounded rationality’. He wrote that humans' decision making can be optimised when they are provided with a limited quantity of relevant, focused information and sufficient time to process it.
With the advent of contemporary AI technologies, the bounds of human rationality have been expanded. AI provides drug researchers with a greater breadth and depth of data that is simultaneously more focused and relevant than the data sets of the past, enabling researchers to optimise their decision-making capacities.
The continued advancement of AI will augment humans’ power of critical thinking in three key areas that are relevant to the medical and pharmaceutical industries: computing advanced mathematical problems, analyzing complex statistics, and generating novel hypotheses. These areas correspond to the ‘three waves’ of AI development throughout the 20th and 21st centuries.
The first and second waves
The first wave of AI development brought us ‘knowledge engineering’ optimisation programs, which solved real-world problems efficiently.
While applications specific to the pharmaceutical industry were scarce, the broader medical field benefits from first-wave AI technologies every day. Take the Framingham Risk Score Calculator, which utilises AI to predict the heart disease risk of any patient.
Machine learning programs were brought along by the second wave of AI. These solve complex pattern recognition problems using statistical analysis. Unlike their first-wave predecessors, second-wave AI programs perceive and learn – often as well as humans do.
Clinical decision support systems use second-wave pattern-recognition programs to analyse and evaluate medical test results. Similar machine-learning programs are beginning to be used by leading pharma firms in a variety of research and development contexts to predict drug effectiveness, to discover new compounds with pharmaceutical qualities, and to develop new combinations of existing drugs.
Second-wave AI is powerful, but it demands well-organised, consistently-coded, and complete data sets in order to accurately conduct its analyses. This limitation is now being overcome by the third wave of AI.
The third wave
We have now entered the third wave of AI development. Third-wave AI programs have the capacity to analyse enormous sets of data, identify patterns, and generate algorithms to explain them. These programs normalise the context of disparate data points and generate original, novel hypotheses at a faster rate and with greater accuracy than human researchers can.
Only in this third wave have AI programs reached a sufficiently advanced state to effectively analyse the vast and complex web of unstructured biological data. Until recently, biological data had to be manually cleaned and organised through extensive and costly human effort. Now, AI programs use a combination of machine learning, natural language processing, and text analytics to analyse unstructured data in real-time.
Through context normalisation, third-wave AI technology dramatically increases the quantity of data that can be analysed in the course of the drug discovery and testing process. Furthermore, it enables the simultaneous generation and testing of new hypotheses at a rate that would be impossible without such immense computing power.
Aided by this technology, drug researchers can arrive at a higher quantity of more accurate hypotheses and can test these hypotheses with unprecedented speed. The result is a significantly faster, and less expensive, discovery process, with lower risk and more effective results. Firms such as Pfizer and Johnson & Johnson are employing such methods to great effect.
Given that R&D consumes as much as 20% of pharma firms’ revenues, and that the total price of developing a new drug has ballooned by 250% in the last 30 years, it’s not surprising that firms are eager to embrace third-wave AI as a means of accelerating drug development.
About the author:
Gunjan Bhardwaj is the founder and CEO of Innoplexus, a leader in AI and analytics as a service for life science industries. With a background at Boston Consulting Group and Ernst & Young, he bridges the worlds of AI, consulting, and life science to drive innovation.
Read more:
How AI, blockchain, and big data can reduce drug development costs