Google and DeepMind share work on medical chatbot Med-PaLM
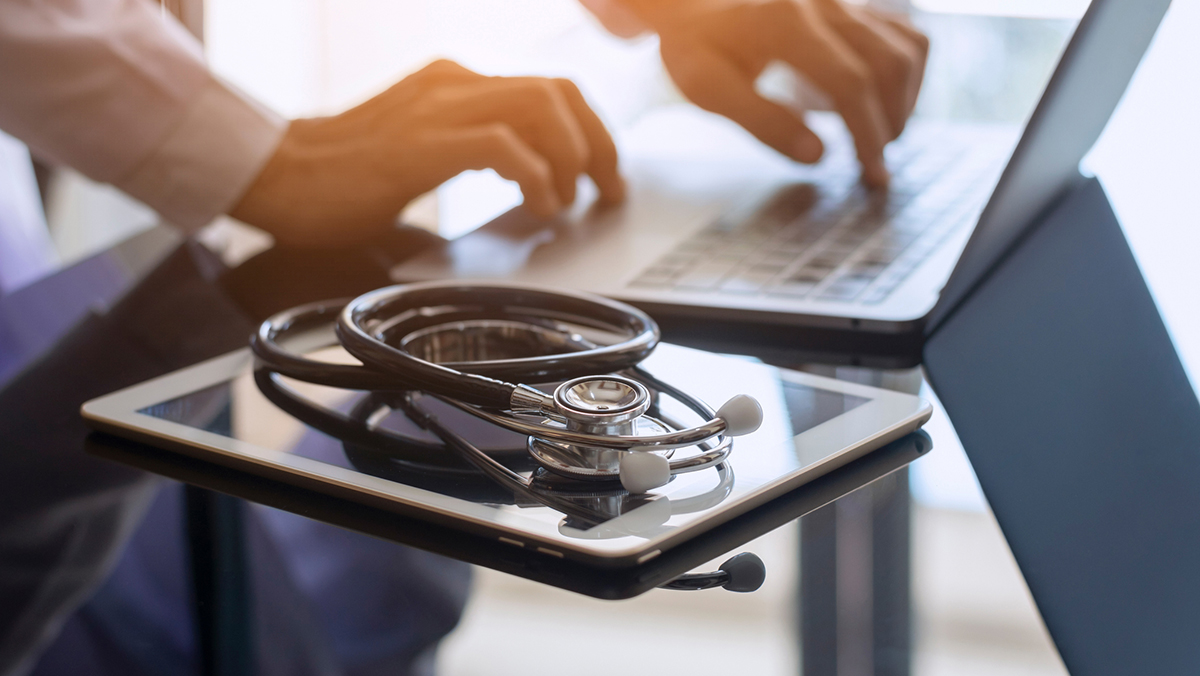
Google and DeepMind have developed an artificial intelligence-powered chatbot tool called Med-PaLM designed to generate "safe and helpful answers" to questions posed by healthcare professionals and patients.
The tool is an example of a large language model or LLM, which are designed to understand queries and generate text responses in plain language, drawing from large and complex datasets – in this case, medical research.
LLMs hit the headlines last year with the launch of OpenAI's ChatGPT, a conversational AI trained on data scraped from the Internet that aims to provide near human interactions, impressing with its ability to answer questions on a wide range of topics, and generate text content on demand, like poems and essays.
It rapidly passed a million users – albeit, with the numbers likely inflated by those trying to entice the chatbot into making scurrilous, inappropriate, or taboo pronouncements.
While ChatGPT is a showcase technology that operates at the consumer end of the LLM scale, Med-PaLM is designed to operate within narrower parameters, and has been trained on seven question-answering datasets spanning professional medical exams, research, and consumer queries about medical matters.
The researchers have published a paper on the LLM, which suggests that with refinement it could have a role to play in clinical applications.
https://twitter.com/AziziShekoofeh/status/1607798892892164096
Six of those datasets are already established (NedQA, MedMCQA, PubMedQA, LiveQA, MedicationQA, and MMLU), but the Google and DeepMind teams have developed their own, called HealthSearchQA, which was curated using questions about medical conditions and their associated symptoms posted online.
The researchers behind the project point to a number of possible applications, including knowledge retrieval, clinical decision support, summarisation of key findings in studies, and triaging patients' primary care concerns, but acknowledge that for now it "performs encouragingly, but remains inferior to clinicians."
For example, incorrect retrieval of information was seen in 16.9% of Med-PaLM responses, compared to less than 4% for human clinicians, according to the paper. There were similar disparities on incorrect reasoning (around 10% versus 2%) and inappropriate or incorrect content of responses (18.7% vs 1.4%).
More important than the results to date are the techniques that can be used to improve that LLM performance, such as the use of instruction prompt tuning, using interaction examples to produce answers that are more helpful to users, according to the team.
Instruction prompt tuning has allowed Med-PaLM to outperform another LLM called Flan-PaLM, with a panel of clinicians judging that 62% of Flan-PaLM long-form answers were accurate, compared to 93% for Med-PaLM.
"Our research provides a glimpse into the opportunities and the challenges of applying these technologies to medicine," write the researchers.
"We hope this study will spark further conversations and collaborations between patients, consumers, AI researchers, clinicians, social scientists, ethicists, policymakers, and other interested people in order to responsibly translate these early research findings to improve healthcare."