Solving the hit-to-lead selection challenge for novel targets
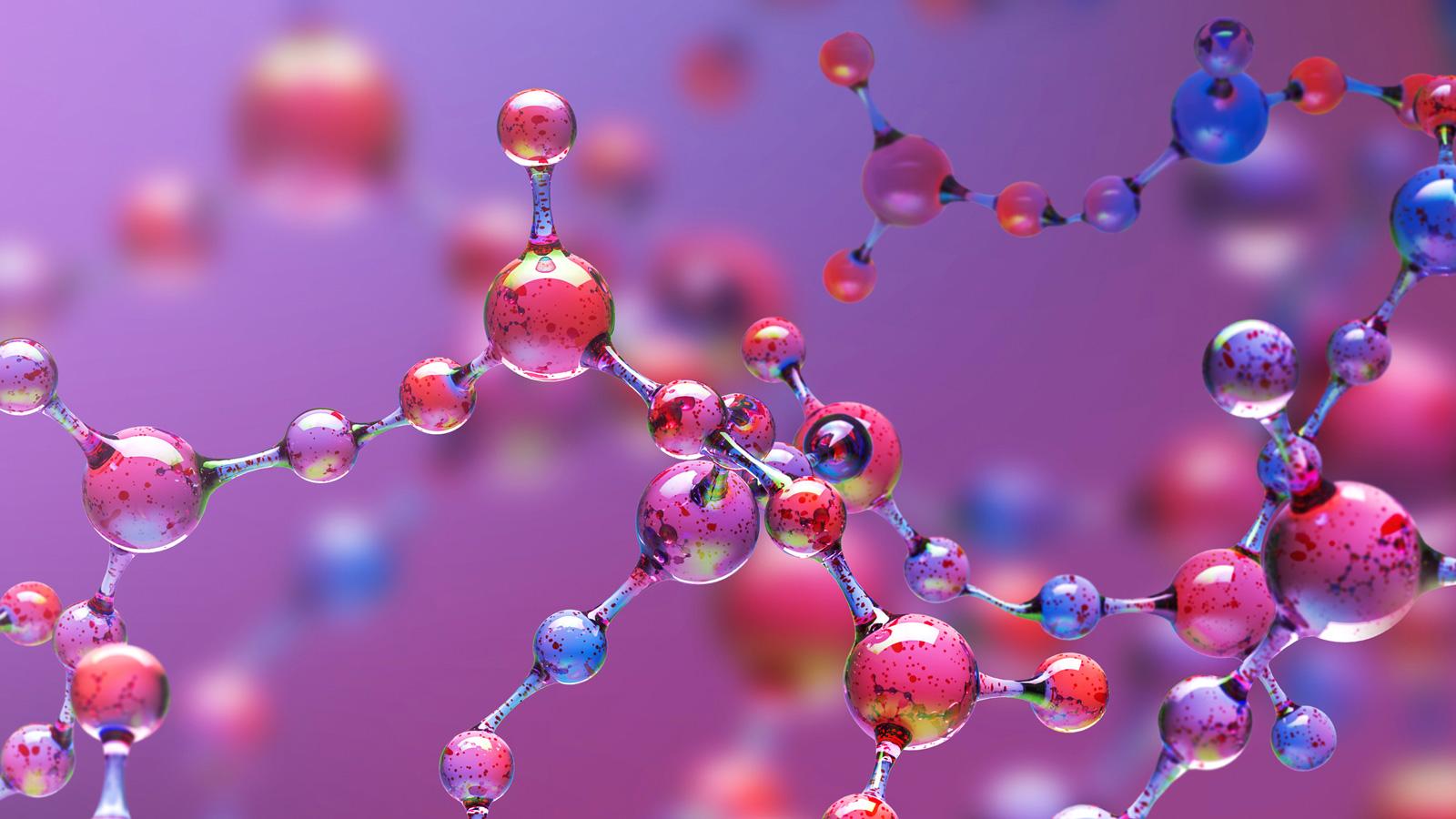
The drug target universe is expanding to include RNA and protein-protein interactions, but poor-quality data at the hit-to-lead and lead optimisation stages is holding back progress. There is an urgent need for new, scalable technologies that can provide dynamic, direct measurements of drug-target interactions to unlock the potential of these untapped therapeutic areas.
The pharmaceutical industry has conventionally focused much of its attention on the development of small molecule therapeutics that inhibit or alter the function of a target protein. However, this approach relies on finding targets that have a suitable drug binding pocket - such as receptors, enzymes, or ion channels - and that binding of the ligand has the desired biological effect with minimal off-target impacts.
Approximately 2,500 human proteins are thought to be associated with disease processes, but fewer than a third can be targeted using currently approved drugs, with much of the remainder deemed ‘undruggable’ using this approach. Furthermore, proteins are not the only biologically active molecules within cells, with five times as many transcribed RNAs in the human transcriptome compared with the expressed proteome.
In recent years, new insights, technologies, and therapeutic modalities have expanded the universe of possible drug targets to include protein-protein interactions and RNA itself. There is significant value to be generated for biopharma companies and patients by tapping into these challenging but largely unexplored targets, but unlocking it is not as easy as it first appears.
Expanding the drug target universe
Targeting protein-protein interactions
Protein-protein interactions (PPIs) are a fundamental component of the machinery of life, with an estimated 130,000 to 650,000 different PPIs in the human interactome. Drugging these interactions - whether to inhibit or stabilise them – is, therefore, a highly attractive therapeutic approach.
A handful of PPI drugs came onto the market in 2014, but little progress has been seen since this initial surge. This is primarily due to a number of technical limitations. Unlike conventional drug targets, which have a well-defined binding site, the interface between interacting proteins is relatively large and featureless, and there are no endogenous ligands to use as a model.
Improvements in sequence and structural analysis, fuelled by artificial intelligence (AI), are helping to better define PPI targets - for example, by identifying key amino acid ‘hot spots’ that are particularly important within the protein interface.
Adding to the challenge, therapeutics targeting PPIs are harder to study than drugs that bind to a single protein target. This requires techniques capable of studying the proteins as they are interacting and observing the impact of a lead molecule on that interaction.
RNA-targeted drug development
Much of the human genome is made up of sequences that are transcribed into non-coding RNAs, which are increasingly recognised as playing a significant role in health and disease. Bacterial and viral RNAs also represent an exciting class of novel antimicrobial targets.
The complex secondary and tertiary structures of RNA allow it to selectively interact with a wide range of small molecules, including chemical compounds, metabolites, and proteins. However, the field of RNA-targeted drug discovery has moved relatively slowly since the first small molecule drug targeting RNA, Evrysdi (risdiplam), was approved for the treatment of spinal muscular atrophy (SMA) in 2020.
Progress is currently hindered by the lack of detailed understanding of RNA conformational dynamics and RNA-drug interactions. This has led to a high false positive rate during hit-to-lead characterisation and lead optimisation stages, followed by subsequent withdrawals during clinical development. Moreover, the risks of off-pathway effects have remained largely unstudied.
Methods for studying molecular interactions are limited
There are several existing technologies for studying drug-target interactions. While each has its own advantages, there are also drawbacks that limit their use for studying the dynamic interactions between these ‘undruggable’ targets and potential novel therapeutics aimed at them.
These limitations leave biopharma companies with an incomplete picture of what is happening as drugs bind to their targets. For example, they can be:
- Indirect, relying on surrogate measurements, rather than direct read-outs of drug-target interactions. For example, liquid chromatography with tandem mass spectrometry (LC-MS/MS), or isothermal titration calorimetry.
- Static, providing a fixed snapshot of a drug bound to a target, rather than measuring dynamic binding kinetics. For example, X-ray crystallography, or fluorescence-based microscopy.
- Unrepresentative, producing an average over thousands of molecules, rather than individual measurements. For example, surface plasmon resonance (SPR), or microscale thermophoresis.
- Inefficient, failing to deliver insights on the scale demanded by modern high-throughput drug discovery pipelines. For example, nuclear magnetic resonance (NMR), atomic force microscopy (AFM), or optical tweezers.
A lack of detailed information about mechanism of action, off-target effects, and binding kinetics is particularly relevant at the hit-to-lead and lead optimisation stage. This results in the selection and progression of sub-optimal lead compounds through the drug development pipeline.
Improving hit-to-lead selection for ‘undruggable’ targets
There is an urgent need for novel technologies that can capture the true nature of molecular interactions between lead compounds and ‘undruggable’ targets like PPIs and RNA, and one example of such is Depixus’MAGNA, a scalable technology for probing molecular interactions with a wide range of applications across the drug discovery pipeline, based on magnetic force spectroscopy.
Having access to detailed information like direct, real-time measurement of dynamic interactions across thousands of individual molecules, could have a significant impact on drug discovery timelines for PPI- and RNA-targeted therapeutics. In turn, this could improve the efficiency of hit-to-lead selection, failing poor compounds at an earlier stage and, ultimately, bringing more effective therapeutics to market faster.